[
Ed. note: This article comes from
a Tweet first posted here
. We’ve partnered with Cameron Wolfe to give his insights a wider audience, as we think they provide insight into various topics around GenAI.
]
Mixture of experts (MoE) has arisen as a new technique to improve LLM performance. With the release of
Grok-1
and the continued popularity of Mistral’s MoE model, this is a good time to take a look at what this technique does.
When applied to transformer models, MoE layers have two primary components:
- Sparse MoE Layer
: replaces dense feed-forward layers in the transformer with a sparse layer of several, similarly-structured “experts”.
- Router
: determines which tokens in the MoE layer are sent to which experts.
Each expert in the sparse MoE layer is just a feed-forward neural network with its own independent set of parameters. The architecture of each expert mimics the feed-forward sub-layer used in the standard transformer architecture. The router takes each token as input and produces a probability distribution over experts that determines to which expert each token is sent. In this way, we drastically increase the model’s capacity (or number of parameters) but avoid excessive computational costs by only using a portion of the experts in each forward pass.
Decoder-only architecture.
Nearly all autoregressive large language models (LLMs) use a decoder-only transformer architecture. This architecture is composed of transformer blocks that contain masked self-attention and feed-forward sub-layers. MoEs modify the feed-forward sub-layer within the transformer block, which is just a feed-forward neural network through which every token within the layer’s input is passed.
Creating an MoE layer.
In an MoE-based LLM, each feed-forward sub-layer in the decoder-only transformer is replaced with an MoE layer. This MoE layer is comprised of several experts, where each of these experts is a feed-forward neural network?
having a similar architecture to the original feed-forward sub-layer
?with an independent set of parameters. We can have anywhere for a few experts to thousands of experts. Grok in particular has eight experts in each MoE layer
Sparse activation.
The MoE model has multiple independent neural networks (i.e., instead of a single feed-forward neural network) within each feed-forward sub-layer of the transformer. However,
we only use a small portion of each MoE layer’s experts in the forward pass
! Given a list of tokens as input, we use a routing mechanism to sparsely select a set of experts to which each token will be sent. For this reason, the computational cost of an MoE model’s forward pass is much less than that of a dense model with the same number of parameters.
How does routing work?
The routing mechanism used by most MoEs is a simple softmax gating function. We pass each token vector through a linear layer that produces an output of size N (i.e., the number of experts), then apply a softmax transformation to convert this output into a probability distribution over experts. From here, we compute the output of the MoE layer:
- Selecting the top-K experts (i.e., k=2 for Grok)
- Scaling the output of each expert by the probability assigned to it by the router
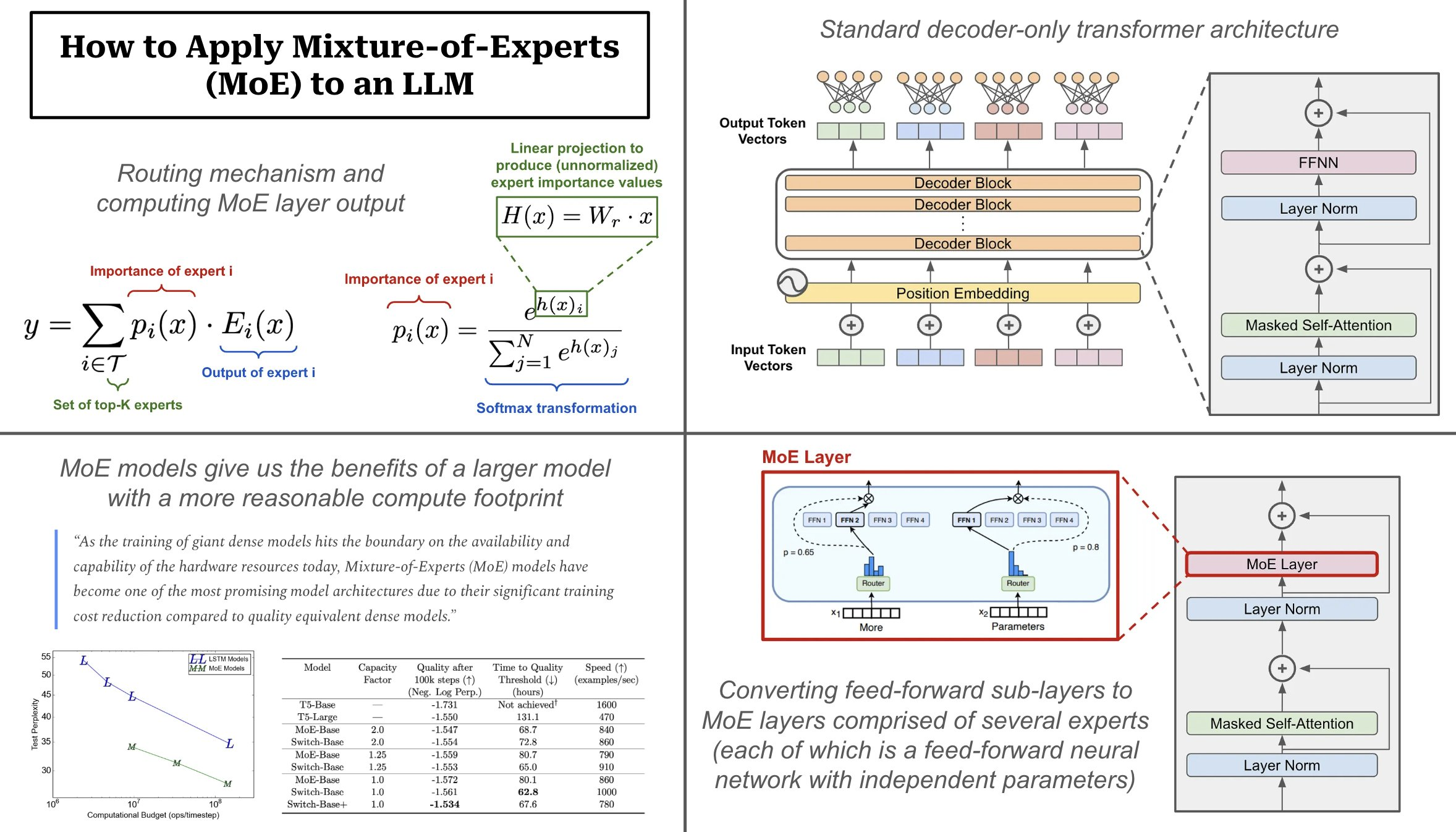
Why are MoEs popular for LLMs?
Increasing the size and capacity of a language model is one of the primary avenues of improving performance, assuming that we have access to a sufficiently large training dataset. However, the cost of training increases with the size of the underlying model, which makes larger models burdensome in practice. MoE models avoid this expense by only using a subset of the model’s parameters during inference. For example, Grok-1 has 314B parameters in total, but only 25% of these parameters are active for a given token.
If you're interested in looking more into the practical/implementation side of MoEs,
check out the OpenMoE project
! It's the best resources on MoEs out there by far (at least in my opinion). OpenMoE is in JAX, but there's also a PyTorch port as well.
Check out
this paper
where we observe interesting scaling laws for MoEs.